Creating a betting model may sound intimidating, but it’s one of the most effective ways to gain an analytical edge over casual bettors. A betting model is essentially a system or formula that uses data and statistics to estimate the probability of an event’s outcome. With that estimation, you can compare your predicted odds to the bookmaker’s and identify potential value bets. Rather than relying on instinct or public opinion, a personal model helps guide your decisions with consistent logic and quantitative reasoning. In this article, we’ll walk you through the fundamentals of building your own betting model—from choosing a sport to collecting data, applying statistical tools, and refining predictions. Whether you’re new to betting analytics or looking to level up your strategy, this guide will help you take the first steps toward smarter, more calculated wagers.
Step 1: Choose Your Sport and Market
The first step is to narrow your focus. Trying to build a universal model for all sports is unrealistic and inefficient. Choose a sport you follow closely and understand well—such as football, basketball, or tennis. Then pick a specific market, like match winners, over/under totals, or point spreads.
Focusing on one sport and market helps you:
- Gather relevant data more efficiently
- Understand the variables that affect outcomes
- Create a more targeted and effective model
For example, if you choose football and want to predict over/under 2.5 goals, your model needs to analyze team offensive and defensive stats, recent form, weather, and more. Precision comes from depth, not breadth—start small and expand once your model shows promise.
Step 2: Collect and Clean Your Data
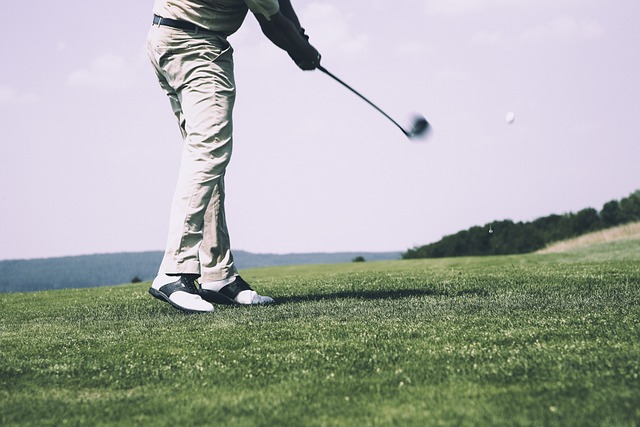
Reliable data is the foundation of any betting model. You’ll need historical data on the teams, players, outcomes, and relevant statistics. Sources include public APIs, sports databases, or manually scraped websites. The more detailed and consistent the data, the more accurate your model can be.
Key data points may include:
- Match results
- Team and player statistics
- Odds history from sportsbooks
- Game location, injuries, and weather conditions
Once you have the data, clean it. That means removing duplicates, correcting errors, standardizing formats, and dealing with missing values. A model is only as good as the quality of the data it relies on.
Step 3: Choose a Modeling Method
There are various statistical techniques for building a betting model. For beginners, logistic regression is a simple yet powerful starting point, especially for binary outcomes (win/loss). More advanced bettors may explore Poisson distribution models, machine learning algorithms, or Elo ratings for dynamic power rankings.
Whatever method you choose, the goal is to:
- Estimate the probability of each outcome
- Convert those probabilities into “fair odds”
- Compare your odds to the bookmaker’s to find value
For example, if your model gives a team a 60% chance of winning, the fair odds would be 1.67. If a sportsbook is offering 1.80, you have a potential value bet.
Step 4: Test, Refine, and Adjust
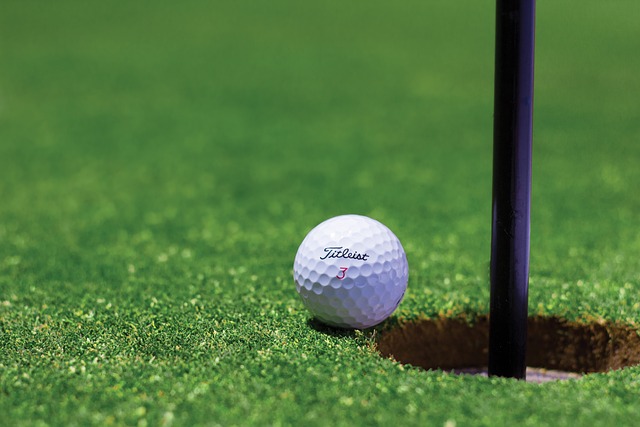
Once you’ve built your model, it’s time to test it on historical data to see how it would have performed. This is called backtesting. Evaluate your model’s success not just by how many bets it “won,” but by whether it beat the closing lineand showed positive expected value (EV) over time.
If your results are poor:
- Re-examine your inputs
- Adjust weightings of certain variables
- Consider additional data you may have missed
Model building is an iterative process. No model is perfect, and even successful ones require constant fine-tuning. As markets evolve and data grows, so should your model.
Conclusion: Build, Bet, Improve
Building your own betting model takes time, patience, and a willingness to learn. But the rewards are worth it—having a custom-built system based on logic and data can give you a sustainable edge in a competitive market. Start by focusing on one sport, gather clean and relevant data, apply the right statistical tools, and continuously refine your approach.
While no model guarantees profit, it does offer structure, discipline, and a repeatable process that can outperform gut instinct and guesswork. In the long run, it’s not about predicting every outcome correctly—it’s about identifying value consistently and managing risk wisely.